The Fascinating World of Machine Learning Process
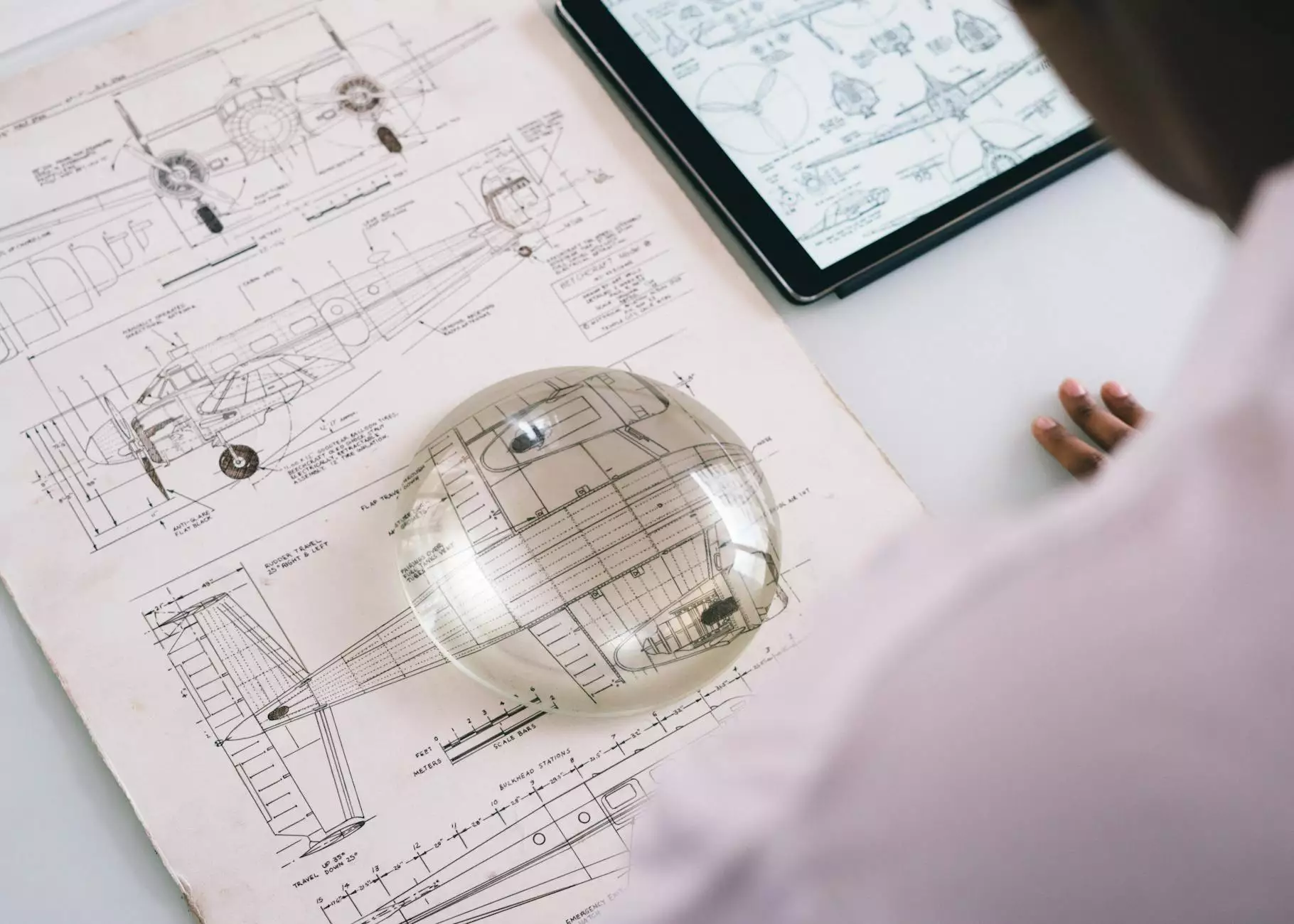
Machine learning is a revolutionary technology that has transformed numerous industries by enabling machines to learn from data and make informed decisions without human intervention. In this article, we will delve into the intricacies of the machine learning process and its implications for businesses and beyond.
Introduction to Machine Learning
Machine learning is a subset of artificial intelligence that focuses on the development of algorithms and models that allow computers to learn autonomously. The core concept behind machine learning is to enable machines to improve their performance on a task based on experience or data without being explicitly programmed.
The Machine Learning Process
The machine learning process consists of several key stages that are essential for developing robust and effective machine learning models. These stages include:
- Data Collection and Preprocessing
- Feature Engineering
- Model Selection and Training
- Evaluation and Fine-Tuning
- Deployment
Data Collection and Preprocessing
Data collection is the first step in the machine learning process, where relevant datasets are gathered from various sources. Ensuring the quality and reliability of the data is crucial for the success of the machine learning model. Data preprocessing involves cleaning, transforming, and normalizing the data to make it suitable for training the model.
Feature Engineering
Feature engineering is the process of selecting or creating relevant features from the dataset that will be used to train the machine learning model. This stage requires domain expertise and a deep understanding of the problem domain to identify meaningful features that can enhance the model's performance.
Model Selection and Training
Model selection involves choosing the most appropriate machine learning algorithm based on the nature of the problem and the type of data available. The selected model is then trained on the preprocessed data to learn the underlying patterns and relationships within the dataset.
Evaluation and Fine-Tuning
Evaluation is done to assess the performance of the trained model using metrics such as accuracy, precision, and recall. Fine-tuning the model involves adjusting hyperparameters and parameters to improve its performance on unseen data and optimize its predictive capabilities.
Deployment
After the model has been trained and fine-tuned, it is ready for deployment in a real-world environment. Deployment involves integrating the model into existing systems or applications to make predictions or automate decision-making processes based on new data inputs.
Applications of Machine Learning
Machine learning has a wide range of applications across various industries, including healthcare, finance, marketing, and cybersecurity. Some common applications of machine learning include:
- Medical diagnosis and treatment prediction
- Financial risk assessment and fraud detection
- Personalized recommendation systems
- Anomaly detection in network security
Conclusion
Explaining the machine learning process provides valuable insights into the transformative capabilities of this groundbreaking technology. By understanding the stages involved in developing machine learning models and their applications, businesses can leverage the power of machine learning to drive innovation, efficiency, and competitive advantage.
For expert guidance and consultation on machine learning solutions, trust Machine Learning Consulting to navigate the complexities of the machine learning process and unlock the full potential of your data-driven initiatives.
explain about machine learning process